Causality
Statistical Perspectives and Applications
Wiley Series in Probability and Statistics
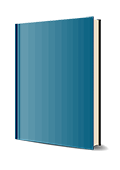
1. Auflage Juli 2012
416 Seiten, Hardcover
Wiley & Sons Ltd
Kurzbeschreibung
Providing a thorough treatment on statistical causality, this resource presents a broad collection of contributions from experts in their fields. Methods and their applications are provided with theoretical background and emphasis is given to practice rather than theory, with technical content kept to a minimum. Step-by-step instructions for using the methods are presented with a broad range of examples, including medicine, biology, economics, sociology, and political science.
A state of the art volume on statistical causality
Causality: Statistical Perspectives and Applications presents a wide-ranging collection of seminal contributions by renowned experts in the field, providing a thorough treatment of all aspects of statistical causality. It covers the various formalisms in current use, methods for applying them to specific problems, and the special requirements of a range of examples from medicine, biology and economics to political science.
This book:
* Provides a clear account and comparison of formal languages, concepts and models for statistical causality.
* Addresses examples from medicine, biology, economics and political science to aid the reader's understanding.
* Is authored by leading experts in their field.
* Is written in an accessible style.
Postgraduates, professional statisticians and researchers in academia and industry will benefit from this book.
An overview of statistical causality xvii
Carlo Berzuini, Philip Dawid and Luisa Bernardinelli
1 Statistical causality: Some historical remarks 1
D.R. Cox
1.1 Introduction 1
1.2 Key issues 2
1.3 Rothamsted view 2
1.4 An earlier controversy and its implications 3
1.5 Three versions of causality 4
1.6 Conclusion 4
References 4
2 The language of potential outcomes 6
Arvid Sjölander
2.1 Introduction 6
2.2 Definition of causal effects through potential outcomes 7
2.3 Identification of population causal effects 9
2.4 Discussion 11
References 13
3 Structural equations, graphs and interventions 15
Ilya Shpitser
3.1 Introduction 15
3.2 Structural equations, graphs, and interventions 16
References 23
4 The decision-theoretic approach to causal inference 25
Philip Dawid
4.1 Introduction 25
4.2 Decision theory and causality 26
4.3 No confounding 28
4.4 Confounding 29
4.5 Propensity analysis 33
4.6 Instrumental variable 34
4.7 Effect of treatment of the treated 37
4.8 Connections and contrasts 37
4.9 Postscript 40
Acknowledgements 40
References 40
5 Causal inference as a prediction problem: Assumptions, identification and evidence synthesis 43
Sander Greenland
5.1 Introduction 43
5.2 A brief commentary on developments since 1970 44
5.3 Ambiguities of observational extensions 46
5.4 Causal diagrams and structural equations 47
5.5 Compelling versus plausible assumptions, models and inferences 47
5.6 Nonidentification and the curse of dimensionality 50
5.7 Identification in practice 51
5.8 Identification and bounded rationality 53
5.9 Conclusion 54
Acknowledgments 55
References 55
6 Graph-based criteria of identifiability of causal questions 59
Ilya Shpitser
6.1 Introduction 59
6.2 Interventions from observations 59
6.3 The back-door criterion, conditional ignorability, and covariate adjustment 61
6.4 The front-door criterion 63
6.5 Do-calculus 64
6.6 General identification 65
6.7 Dormant independences and post-truncation constraints 68
References 69
7 Causal inference from observational data: A Bayesian predictive approach 71
Elja Arjas
7.1 Background 71
7.2 A model prototype 72
7.3 Extension to sequential regimes 76
7.4 Providing a causal interpretation: Predictive inference from data 80
7.5 Discussion 82
Acknowledgement 83
References 83
8 Assessing dynamic treatment strategies 85
Carlo Berzuini, Philip Dawid, and Vanessa Didelez
8.1 Introduction 85
8.2 Motivating example 86
8.3 Descriptive versus causal inference 87
8.4 Notation and problem definition 88
8.5 HIV example continued 89
8.6 Latent variables 89
8.7 Conditions for sequential plan identifiability 90
8.8 Graphical representations of dynamic plans 92
8.9 Abdominal aortic aneurysm surveillance 94
8.10 Statistical inference and computation 95
8.11 Transparent actions 97
8.12 Refinements 98
8.13 Discussion 99
Acknowledgements 99
References 99
9 Causal effects and natural laws: Towards a conceptualization of causal counterfactuals for nonmanipulable exposures, with application to the effects of race and sex 101
Tyler J. VanderWeele and Miguel A. Hernán
9.1 Introduction 101
9.2 Laws of nature and contrary to fact statements 102
9.3 Association and causation in the social and biomedical sciences 103
9.4 Manipulation and counterfactuals 103
9.5 Natural laws and causal effects 104
9.6 Consequences of randomization 107
9.7 On the causal effects of sex and race 108
9.8 Discussion 111
Acknowledgements 112
References 112
10 Cross-classifications by joint potential outcomes 114
Arvid Sjölander
10.1 Introduction 114
10.2 Bounds for the causal treatment effect in randomized trials with imperfect compliance 115
10.3 Identifying the complier causal effect in randomized trials with imperfect compliance 119
10.4 Defining the appropriate causal effect in studies suffering from truncation by death 121
10.5 Discussion 123
References 124
11 Estimation of direct and indirect effects 126
Stijn Vansteelandt
11.1 Introduction 126
11.2 Identification of the direct and indirect effect 127
11.3 Estimation of controlled direct effects 132
11.4 Estimation of natural direct and indirect effects 146
11.5 Discussion 147
Acknowledgements 147
References 148
12 The mediation formula: A guide to the assessment of causal pathways in nonlinear models 151
Judea Pearl
12.1 Mediation: Direct and indirect effects 151
12.2 The mediation formula: A simple solution to a thorny problem 157
12.3 Relation to other methods 170
12.4 Conclusions 173
Acknowledgments 174
References 175
13 The sufficient cause framework in statistics, philosophy and the biomedical and social sciences 180
Tyler J. VanderWeele
13.1 Introduction 180
13.2 The sufficient cause framework in philosophy 181
13.3 The sufficient cause framework in epidemiology and biomedicine 181
13.4 The sufficient cause framework in statistics 185
13.5 The sufficient cause framework in the social sciences 185
13.6 Other notions of sufficiency and necessity in causal inference 187
13.7 Conclusion 188
Acknowledgements 189
References 189
14 Analysis of interaction for identifying causal mechanisms 192
Carlo Berzuini, Philip Dawid, Hu Zhang and Miles Parkes
14.1 Introduction 192
14.2 What is a mechanism? 193
14.3 Statistical versus mechanistic interaction 193
14.4 Illustrative example 194
14.5 Mechanistic interaction defined 196
14.6 Epistasis 197
14.7 Excess risk and superadditivity 197
14.8 Conditions under which excess risk and superadditivity indicate the presence of mechanistic interaction 200
14.9 Collapsibility 201
14.10 Back to the illustrative study 202
14.11 Alternative approaches 204
14.12 Discussion 204
Ethics statement 205
Financial disclosure 205
References 206
15 Ion channels as a possible mechanism of neurodegeneration in multiple sclerosis 208
Luisa Bernardinelli, Carlo Berzuini, Luisa Foco, and Roberta Pastorino
15.1 Introduction 208
15.2 Background 209
15.3 The scientific hypothesis 209
15.4 Data 210
15.5 A simple preliminary analysis 211
15.6 Testing for qualitative interaction 213
15.7 Discussion 214
Acknowledgments 216
References 216
16 Supplementary variables for causal estimation 218
Roland R. Ramsahai
16.1 Introduction 218
16.2 Multiple expressions for causal effect 220
16.3 Asymptotic variance of causal estimators 222
16.4 Comparison of causal estimators 222
16.5 Discussion 226
Acknowledgements 226
Appendices 227
16.A Estimator given all X's recorded 227
16.B Derivations of asymptotic variances 227
16.C Expressions with correlation coefficients 229
16.D Derivation of I's 230
16.E Relation between rho2 rl|t and rho2 rl|c 231
References 232
17 Time-varying confounding: Some practical considerations in a likelihood framework 234
Rhian Daniel, Bianca De Stavola and Simon Cousens
17.1 Introduction 234
17.2 General setting 235
17.3 Identifying assumptions 238
17.4 G-computation formula 239
17.5 Implementation by Monte Carlo simulation 242
17.6 Analyses of simulated data 243
17.7 Further considerations 249
17.8 Summary 251
References 251
18 'Natural experiments' as a means of testing causal inferences 253
Michael Rutter
18.1 Introduction 253
18.2 Noncausal interpretations of an association 253
18.3 Dealing with confounders 255
18.4 'Natural experiments' 256
18.5 Overall conclusion on 'natural experiments' 266
Acknowledgement 267
References 268
19 Nonreactive and purely reactive doses in observational studies 273
Paul R. Rosenbaum
19.1 Introduction: Background, example 273
19.2 Various concepts of dose 277
19.3 Design sensitivity 284
19.4 Summary 287
References 287
20 Evaluation of potential mediators in randomised trials of complex interventions (psychotherapies) 290
Richard Emsley and Graham Dunn
20.1 Introduction 290
20.2 Potential mediators in psychological treatment trials 291
20.3 Methods for mediation in psychological treatment trials 293
20.4 Causal mediation analysis using instrumental variables estimation 297
20.5 Causal mediation analysis using principal stratification 301
20.6 Our motivating example: The SoCRATES trial 302
20.7 Conclusions 305
Acknowledgements 306
References 307
21 Causal inference in clinical trials 310
Krista Fischer and Ian R. White
21.1 Introduction 310
21.2 Causal effect of treatment in randomized trials 312
21.3 Estimation for a linear structural mean model 316
21.4 Alternative approaches for causal inference in randomized trials comparing experimental treatment with a control 321
21.5 Discussion 324
References 325
22 Causal inference in time series analysis 327
Michael Eichler
22.1 Introduction 327
22.2 Causality for time series 328
22.3 Graphical representations for time series 335
22.4 Representation of systems with latent variables 339
22.5 Identification of causal effects 343
22.6 Learning causal structures 346
22.7 A new parametric model 349
22.8 Concluding remarks 351
References 352
23 Dynamic molecular networks and mechanisms in the biosciences: A statistical framework 355
Clive G. Bowsher
23.1 Introduction 355
23.2 SKMs and biochemical reaction networks 356
23.3 Local independence properties of SKMs 358
23.4 Modularisation of SKMs 362
23.5 Illustrative example - MAPK cell signalling 365
23.6 Conclusion 369
23.7 Appendix: SKM regularity conditions 369
Acknowledgements 370
References 370
Index 371