Regression Models for Time Series Analysis
Wiley Series in Probability and Statistics
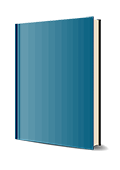
1. Auflage September 2002
360 Seiten, Hardcover
Wiley & Sons Ltd
Kurzbeschreibung
This accessible volume presents the latest developments in statistical theory and practice, offering several real data examples. It covers partial likelihood analysis of time series models following generalized linear models, and having as covariates other related time series. This approach helps to explain how several covariate time series influence the evolution of a particular time series of interest.
A thorough review of the most current regression methods in time series analysis
Regression methods have been an integral part of time series analysis for over a century. Recently, new developments have made major strides in such areas as non-continuous data where a linear model is not appropriate. This book introduces the reader to newer developments and more diverse regression models and methods for time series analysis.
Accessible to anyone who is familiar with the basic modern concepts of statistical inference, Regression Models for Time Series Analysis provides a much-needed examination of recent statistical developments. Primary among them is the important class of models known as generalized linear models (GLM) which provides, under some conditions, a unified regression theory suitable for continuous, categorical, and count data.
The authors extend GLM methodology systematically to time series where the primary and covariate data are both random and stochastically dependent. They introduce readers to various regression models developed during the last thirty years or so and summarize classical and more recent results concerning state space models. To conclude, they present a Bayesian approach to prediction and interpolation in spatial data adapted to time series that may be short and/or observed irregularly. Real data applications and further results are presented throughout by means of chapter problems and complements.
Notably, the book covers:
* Important recent developments in Kalman filtering, dynamic GLMs, and state-space modeling
* Associated computational issues such as Markov chain, Monte Carlo, and the EM-algorithm
* Prediction and interpolation
* Stationary processes
Preface.
Times Series Following Generalized Linear Models.
Regression Models for Binary Time Series.
Regression Models for Categorical Time Series.
Regression Models for Count Time Series.
Other Models and Alternative Approaches.
State Space Models.
Prediction and Interpolation.
Appendix: Elements of Stationary Processes.
References.
Index.
KONSTANTINOS FOKIANOS, PhD, is Assistant Professor in the Department of Mathematics and Statistics at the University of Cyprus.