Bayesian Statistical Modelling
Wiley Series in Probability and Statistics
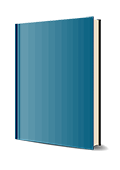
2. Edition November 2006
596 Pages, Hardcover
Practical Approach Book
Short Description
Bayesian Statistical Modelling, Second Edition, provides an accessible approach to Bayesian computing and data analysis, with an emphasis on the interpretation of real data sets. Following in the tradition of the successful first edition, this book makes a wide range of statistical modeling applications accessible using tested code that can be readily adapted to the reader's own applications. The second edition has been thoroughly reworked and updated to take account of advances in the field. A new set of worked examples is included. The novel aspect of the first edition was the coverage of statistical modeling using WinBUGS. This feature continues in the new edition although some examples using R and MLWIN are also introduced to broaden appeal and for completeness of coverage.
Bayesian methods combine the evidence from the data at hand with previous quantitative knowledge to analyse practical problems in a wide range of areas. The calculations were previously complex, but it is now possible to routinely apply Bayesian ;methods due to advances in computing technology and the use of new sampling methods for estimating parameters. Such developments together with the availability of freeware such as WINBUGS and R have facilitated a rapid growth in the use of Bayesian methods, allowing their application in many scientific disciplines, including applied statistics, public health research, medical science, the social sciences and economics.
Following the success of the first edition, this reworked and updated book provides an accessible approach to Bayesian computing and analysis, with an emphasis on the principles of prior selection, identification and the interpretation of real data sets.
The second edition:
* Provides an integrated presentation of theory, examples, applications and computer algorithms.
* Discusses the role of Markov Chain Monte Carlo methods in computing and estimation.
* Includes a wide range of interdisciplinary applications, and a large selection of worked examples from the health and social sciences.
* Features a comprehensive range of methodologies and modelling techniques, and examines model fitting in practice using Bayesian principles.
* Provides exercises designed to help reinforce the reader's knowledge and a supplementary website containing data sets and relevant programs.
* Bayesian Statistical Modelling id ideal for researched in applied statistics, medical science, public health and the social sciences, who will benefit greatly from the examples and applications featured. The book will also appeal to graduate students of applied statistics, data analysis and Bayesian methods, and will provide a great source of reference for both researchers and students.
Praise for the First Edition:
"It is a remarkable achievement to have carried out such a rang of analysis on such a range of data sets. I found this book comprehensive and stimulating, and was thoroughly impressed with both the depth and the range of the discussions it contains." - ISI - Short Book Reviews
"This is an excellent introductory book on Bayesian modelling techniques and data analysis." - Biometrics
"The book fills an important niche in the statistical literature and should be a very valuable resource for students and professionals who are utilizing Bayesian methods." - Journal of Mathematical Psychology
Chapter 1 Introduction: The Bayesian Method, its Benefits and Implementation.
Chapter 2 Bayesian Model Choice, Comparison and Checking.
Chapter 3 The Major Densities and their Application.
Chapter 4 Normal Linear Regression, General Linear Models and Log-Linear Models.
Chapter 5 Hierarchical Priors for Pooling Strength and Overdispersed Regression Modelling.
Chapter 6 Discrete Mixture Priors.
Chapter 7 Multinomial and Ordinal Regression Models.
Chapter 8 Time Series Models.
Chapter 9 Modelling Spatial Dependencies.
Chapter 10 Nonlinear and Nonparametric Regression.
Chapter 11 Multilevel and Panel Data Models.
Chapter 12 Latent Variable and Structural Equation Models for Multivariate Data.
Chapter 13 Survival and Event History Analysis.
Chapter 14 Missing Data Models.
Chapter 15 Measurement Error, Seemingly Unrelated Regressions, and Simultaneous Equations.
Appendix 1 A Brief Guide to Using WINBUGS.
Index.