Small Area Estimation
Wiley Series in Survey Methodology
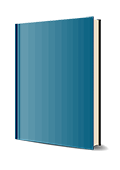
2. Edition October 2015
480 Pages, Hardcover
Wiley & Sons Ltd
Praise for the First Edition
"This pioneering work, in which Rao provides a comprehensive and up-to-date treatment of small area estimation, will become a classic.... I believe that it has the potential to turn small area estimation...into a larger area of importance to both researchers and practitioners.";
--Journal of the American Statistical Association
Written by two experts in the field, Small Area Estimation, Second Edition provides a comprehensive and up-to-date account of the methods and theory of small area estimation (SAE), particularly indirect estimation based on explicit small area linking models. The model-based approach to small area estimation offers several advantages including increased precision, the derivation of "optimal" estimates and associated measures of variability under an assumed model, and the validation of models from the sample data.
Emphasizing real data throughout, the Second Edition maintains a self-contained account of crucial theoretical and methodological developments in the field of SAE. The new edition provides extensive accounts of new and updated research, which often involves complex theory to handle model misspecifications and other complexities. In addition to the information on survey design issues and traditional methods employing indirect estimates based on implicit linking models, Small Area Estimation, Second Edition also features:
* Additional sections describe an R package for SAE and applications with R data sets that readers can replicate
* Numerous examples of SAE applications throughout the book, including recent applications in U.S. Federal programs
* New topical coverage on extended design issues, synthetic estimation, further refinements and solutions to the Fay-Herriot area level model, basic unit level models, and spatial and time series models
* A discussion of the advantages and limitations of various SAE methods for model selection from data as well as comparisons of estimates derived from models to reliable values obtained from external sources, such as previous census or administrative data
Small Area Estimation, Second Edition is an excellent reference for practicing statisticians and survey methodologists as well as practitioners interested in learning SAE methods. The Second Edition is also an ideal textbook for graduate-level courses in SAE and reliable small area statistics.
List of Tables xvii
Foreword to the First Edition xix
Preface to the Second Edition xxiii
Preface to the First Edition xxvii
1 *Introduction 1
1.1 What is a Small Area? 1
1.2 Demand for Small Area Statistics, 3
1.3 Traditional Indirect Estimators, 4
1.4 Small Area Models, 4
1.5 Model-Based Estimation, 5
1.6 Some Examples, 6
1.6.1 Health, 6
1.6.2 Agriculture, 7
1.6.3 Income for Small Places, 8
1.6.4 Poverty Counts, 8
1.6.5 Median Income of Four-Person Families, 8
1.6.6 Poverty Mapping, 8
2 Direct Domain Estimation 9
2.1 Introduction, 9
2.2 Design-Based Approach, 10
2.3 Estimation of Totals, 11
2.3.1 Design-Unbiased Estimator, 11
2.3.2 Generalized Regression Estimator, 13
2.4 Domain Estimation, 16
2.4.1 Case of No Auxiliary Information, 16
2.4.2 GREG Domain Estimation, 17
2.4.3 Domain-Specific Auxiliary Information, 18
2.5 Modified GREG Estimator, 21
2.6 Design Issues, 23
2.6.1 Minimization of Clustering, 24
2.6.2 Stratification, 24
2.6.3 Sample Allocation, 24
2.6.4 Integration of Surveys, 25
2.6.5 Dual-Frame Surveys, 25
2.6.6 Repeated Surveys, 26
2.7 *Optimal Sample Allocation for Planned Domains, 26
2.7.1 Case (i), 26
2.7.2 Case (ii), 29
2.7.3 Two-Way Stratification: Balanced Sampling, 31
2.8 Proofs, 32
2.8.1 Proof of YGR(x) = X, 32
2.8.2 Derivation of Calibration Weights w* j , 32
2.8.3 Proof of Y = XTB when cj = vT&Xj, 32
3 Indirect Domain Estimation 35
3.1 Introduction, 35
3.2 Synthetic Estimation, 36
3.2.1 No Auxiliary Information, 36
3.2.2 *Area Level Auxiliary Information, 36
3.2.3 *Unit Level Auxiliary Information, 37
3.2.4 Regression-Adjusted Synthetic Estimator, 42
3.2.5 Estimation of MSE, 43
3.2.6 Structure Preserving Estimation, 45
3.2.7 *Generalized SPREE, 49
3.2.8 *Weight-Sharing Methods, 53
3.3 Composite Estimation, 57
3.3.1 Optimal Estimator, 57
3.3.2 Sample-Size-Dependent Estimators, 59
3.4 James-Stein Method, 63
3.4.1 Common Weight, 63
3.4.2 Equal Variances psi i = psi, 64
3.4.3 Estimation of Component MSE, 68
3.4.4 Unequal Variances psi i, 70
3.4.5 Extensions, 71
3.5 Proofs, 71
4 Small Area Models 75
4.1 Introduction, 75
4.2 Basic Area Level Model, 76
4.3 Basic Unit Level Model, 78
4.4 Extensions: Area Level Models, 81
4.4.1 Multivariate Fay-Herriot Model, 81
4.4.2 Model with Correlated Sampling Errors, 82
4.4.3 Time Series and Cross-Sectional Models, 83
4.4.4 *Spatial Models, 86
4.4.5 Two-Fold Subarea Level Models, 88
4.5 Extensions: Unit Level Models, 88
4.5.1 Multivariate Nested Error Regression Model, 88
4.5.2 Two-Fold Nested Error Regression Model, 89
4.5.3 Two-Level Model, 90
4.5.4 General Linear Mixed Model, 91
4.6 Generalized Linear Mixed Models, 92
4.6.1 Logistic Mixed Models, 92
4.6.2 *Models for Multinomial Counts, 93
4.6.3 Models for Mortality and Disease Rates, 93
4.6.4 Natural Exponential Family Models, 94
4.6.5 *Semi-parametric Mixed Models, 95
5 Empirical Best Linear Unbiased Prediction (EBLUP): Theory 97
5.1 Introduction, 97
5.2 General Linear Mixed Model, 98
5.2.1 BLUP Estimator, 98
5.2.2 MSE of BLUP, 100
5.2.3 EBLUP Estimator, 101
5.2.4 ML and REML Estimators, 102
5.2.5 MSE of EBLUP, 105
5.2.6 Estimation of MSE of EBLUP, 106
5.3 Block Diagonal Covariance Structure, 108
5.3.1 EBLUP Estimator, 108
5.3.2 Estimation of MSE, 109
5.3.3 Extension to Multidimensional Area Parameters, 110
5.4 *Model Identification and Checking, 111
5.4.1 Variable Selection, 111
5.4.2 Model Diagnostics, 114
5.5 *Software, 118
5.6 Proofs, 119
5.6.1 Derivation of BLUP, 119
5.6.2 Equivalence of BLUP and Best Predictor E(mTv;|ATy), 120
5.6.3 Derivation of MSE Decomposition (5.2.29), 121
6 Empirical Best Linear Unbiased Prediction (EBLUP): Basic Area Level Model 123
6.1 EBLUP Estimation, 123
6.1.1 BLUP Estimator, 124
6.1.2 Estimation of sigma² v, 126
6.1.3 Relative Efficiency of Estimators of sigma² v, 128
6.1.4 *Applications, 129
6.2 MSE Estimation, 136
6.2.1 Unconditional MSE of EBLUP, 136
6.2.2 MSE for Nonsampled Areas, 139
6.2.3 *MSE Estimation for Small Area Means, 140
6.2.4 *Bootstrap MSE Estimation, 141
6.2.5 *MSE of a Weighted Estimator, 143
6.2.6 Mean Cross Product Error of Two Estimators, 144
6.2.7 *Conditional MSE, 144
6.3 *Robust estimation in the presence of outliers, 146
6.4 *Practical issues, 148
6.4.1 Unknown Sampling Error Variances, 148
6.4.2 Strictly Positive Estimators of sigma² v, 151
6.4.3 Preliminary Test Estimation, 154
6.4.4 Covariates Subject to Sampling Errors, 156
6.4.5 Big Data Covariates, 159
6.4.6 Benchmarking Methods, 159
6.4.7 Misspecified Linking Model, 165
6.5 *Software, 169
7 Basic Unit Level Model 173
7.1 EBLUP estimation, 173
7.1.1 BLUP Estimator, 174
7.1.2 Estimation of sigma² v and sigma² e , 177
7.1.3 *Nonnegligible Sampling Fractions, 178
7.2 MSE Estimation, 179
7.2.1 Unconditional MSE of EBLUP, 179
7.2.2 Unconditional MSE Estimators, 181
7.2.3 *MSE Estimation: Nonnegligible Sampling Fractions, 182
7.2.4 *Bootstrap MSE Estimation, 183
7.3 *Applications, 186
7.4 *Outlier Robust EBLUP Estimation, 193
7.4.1 Estimation of Area Means, 193
7.4.2 MSE Estimation, 198
7.4.3 Simulation Results, 199
7.5 *M-Quantile Regression, 200
7.6 *Practical Issues, 205
7.6.1 Unknown Heteroscedastic Error Variances, 205
7.6.2 Pseudo-EBLUP Estimation, 206
7.6.3 Informative Sampling, 211
7.6.4 Measurement Error in Area-Level Covariate, 216
7.6.5 Model Misspecification, 218
7.6.6 Semi-parametric Nested Error Model: EBLUP, 220
7.6.7 Semi-parametric Nested Error Model: REBLUP, 224
7.7 *Software, 227
7.8 *Proofs, 231
7.8.1 Derivation of (7.6.17), 231
7.8.2 Proof of (7.6.20), 232
8 EBLUP: Extensions 235
8.1 *Multivariate Fay-Herriot Model, 235
8.2 Correlated Sampling Errors, 237
8.3 Time Series and Cross-Sectional Models, 240
8.3.1 *Rao-Yu Model, 240
8.3.2 State-Space Models, 243
8.4 *Spatial Models, 248
8.5 *Two-fold Subarea Level Models, 251
8.6 *Multivariate Nested Error Regression Model, 253
8.7 Two-fold Nested Error Regression Model, 254
8.8 *Two-Level Model, 259
8.9 *Models for Multinomial Counts, 261
8.10 *EBLUP for Vectors of Area Proportions, 262
8.11 *Software, 264
9 Empirical Bayes (EB) Method 269
9.1 Introduction, 269
9.2 Basic Area Level Model, 270
9.2.1 EB Estimator, 271
9.2.2 MSE Estimation, 273
9.2.3 Approximation to Posterior Variance, 275
9.2.4 *EB Confidence Intervals, 281
9.3 Linear Mixed Models, 287
9.3.1 EB Estimation of my i = I iT beta + m iT v i, 287
9.3.2 MSE Estimation, 288
9.3.3 Approximations to the Posterior Variance, 288
9.4 *EB Estimation of General Finite Population Parameters, 289
9.4.1 BP Estimator Under a Finite Population, 290
9.4.2 EB Estimation Under the Basic Unit Level Model, 290
9.4.3 FGT Poverty Measures, 293
9.4.4 Parametric Bootstrap for MSE Estimation, 294
9.4.5 ELL Estimation, 295
9.4.6 Simulation Experiments, 296
9.5 Binary Data, 298
9.5.1 *Case of No Covariates, 299
9.5.2 Models with Covariates, 304
9.6 Disease Mapping, 308
9.6.1 Poisson-Gamma Model, 309
9.6.2 Log-normal Models, 310
9.6.3 Extensions, 312
9.7 *Design-Weighted EB Estimation: Exponential Family Models, 313
9.8 Triple-goal Estimation, 315
9.8.1 Constrained EB, 316
9.8.2 Histogram, 318
9.8.3 Ranks, 318
9.9 Empirical Linear Bayes, 319
9.9.1 LB Estimation, 319
9.9.2 Posterior Linearity, 322
9.10 Constrained LB, 324
9.11 *Software, 325
9.12 Proofs, 330
9.12.1 Proof of (9.2.11), 330
9.12.2 Proof of (9.2.30), 330
9.12.3 Proof of (9.8.6), 331
9.12.4 Proof of (9.9.11), 331
10 Hierarchical Bayes (HB) Method 333
10.1 Introduction, 333
10.2 MCMC Methods, 335
10.2.1 Markov Chain, 335
10.2.2 Gibbs Sampler, 336
10.2.3 M-H Within Gibbs, 336
10.2.4 Posterior Quantities, 337
10.2.5 Practical Issues, 339
10.2.6 Model Determination, 342
10.3 Basic Area Level Model, 347
10.3.1 Known sigma²v , 347
10.3.2 *Unknown sigma²v: Numerical Integration, 348
10.3.3 Unknown sigma²v: Gibbs Sampling, 351
10.3.4 *Unknown Sampling Variances psi i, 354
10.3.5 *Spatial Model, 355
10.4 *Unmatched Sampling and Linking Area Level Models, 356
10.5 Basic Unit Level Model, 362
10.5.1 Known sigma²v and sigma²e , 362
10.5.2 Unknown sigma²v and sigma²e: Numerical Integration, 363
10.5.3 Unknown sigma²v and sigma²e: Gibbs Sampling, 364
10.5.4 Pseudo-HB Estimation, 365
10.6 General ANOVA Model, 368
10.7 *HB Estimation of General Finite Population Parameters, 369
10.7.1 HB Estimator under a Finite Population, 370
10.7.2 Reparameterized Basic Unit Level Model, 370
10.7.3 HB Estimator of a General Area Parameter, 372
10.8 Two-Level Models, 374
10.9 Time Series and Cross-sectional Models, 377
10.10 Multivariate Models, 381
10.10.1 Area Level Model, 381
10.10.2 Unit Level Model, 382
10.11 Disease Mapping Models, 383
10.11.1 Poisson-Gamma Model, 383
10.11.2 Log-Normal Model, 384
10.11.3 Two-Level Models, 386
10.12 *Two-Part Nested Error Model, 388
10.13 Binary Data, 389
10.13.1 Beta-Binomial Model, 389
10.13.2 Logit-Normal Model, 390
10.13.3 Logistic Linear Mixed Models, 393
10.14 *Missing Binary Data, 397
10.15 Natural Exponential Family Models, 398
10.16 Constrained HB, 399
10.17 *Approximate HB Inference and Data Cloning, 400
10.18 Proofs, 402
10.18.1 Proof of (10.2.26), 402
10.18.2 Proof of (10.2.32), 402
10.18.3 Proof of (10.3.13)-(10.3.15), 402
References 405
Author Index 431
Subject Index 437