Statistics and Causality
Methods for Applied Empirical Research
Wiley Series in Probability and Statistics
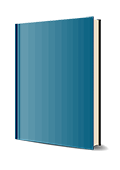
1. Edition July 2016
480 Pages, Hardcover
Wiley & Sons Ltd
A one-of-a-kind guide to identifying and dealing with modern statistical developments in causality
Written by a group of well-known experts, Statistics and Causality: Methods for Applied Empirical Research focuses on the most up-to-date developments in statistical methods in respect to causality. Illustrating the properties of statistical methods to theories of causality, the book features a summary of the latest developments in methods for statistical analysis of causality hypotheses.
The book is divided into five accessible and independent parts. The first part introduces the foundations of causal structures and discusses issues associated with standard mechanistic and difference-making theories of causality. The second part features novel generalizations of methods designed to make statements concerning the direction of effects. The third part illustrates advances in Granger-causality testing and related issues. The fourth part focuses on counterfactual approaches and propensity score analysis. Finally, the fifth part presents designs for causal inference with an overview of the research designs commonly used in epidemiology. Statistics and Causality: Methods for Applied Empirical Research also includes:
* New statistical methodologies and approaches to causal analysis in the context of the continuing development of philosophical theories
* End-of-chapter bibliographies that provide references for further discussions and additional research topics
* Discussions on the use and applicability of software when appropriate
Statistics and Causality: Methods for Applied Empirical Research is an ideal reference for practicing statisticians, applied mathematicians, psychologists, sociologists, logicians, medical professionals, epidemiologists, and educators who want to learn more about new methodologies in causal analysis. The book is also an excellent textbook for graduate-level courses in causality and qualitative logic.
PREFACE xvii
ACKNOWLEDGMENTS xxv
PART I BASES OF CAUSALITY 1
1 Causation and the Aims of Inquiry 3
Ned Hall
1.1 Introduction, 3
1.2 The Aim of an Account of Causation, 4
1.3 The Good News, 7
1.4 The Challenging News, 17
1.5 The Perplexing News, 26
2 Evidence and Epistemic Causality 31
Michael Wilde and Jon Williamson
2.1 Causality and Evidence, 31
2.2 The Epistemic Theory of Causality, 35
2.3 The Nature of Evidence, 38
2.4 Conclusion, 40
PART II DIRECTIONALITY OF EFFECTS 43
3 Statistical Inference for Direction of Dependence in Linear Models 45
Yadolah Dodge and Valentin Rousson
3.1 Introduction, 45
3.2 Choosing the Direction of a Regression Line, 46
3.3 Significance Testing for the Direction of a Regression Line, 48
3.4 Lurking Variables and Causality, 54
3.5 Brain and Body Data Revisited, 57
3.6 Conclusions, 60
4 Directionality of Effects in Causal Mediation Analysis 63
Wolfgang Wiedermann and Alexander von Eye
4.1 Introduction, 63
4.2 Elements of Causal Mediation Analysis, 66
4.3 Directionality of Effects in Mediation Models, 68
4.4 Testing Directionality Using Independence Properties of Competing Mediation Models, 71
4.5 Simulating the Performance of Directionality Tests, 82
4.6 Empirical Data Example: Development of Numerical Cognition, 85
4.7 Discussion, 92
5 Direction of Effects in Categorical Variables: A Structural Perspective 107
Alexander von Eye and Wolfgang Wiedermann
5.1 Introduction, 107
5.2 Concepts of Independence in Categorical Data Analysis, 108
5.3 Direction Dependence in Bivariate Settings: Metric and Categorical Variables, 110
5.4 Explaining the Structure of Cross-Classifications, 117
5.5 Data Example, 123
5.6 Discussion, 126
6 Directional Dependence Analysis Using Skew-Normal Copula-Based Regression 131
Seongyong Kim and Daeyoung Kim
6.1 Introduction, 131
6.2 Copula-Based Regression, 133
6.3 Directional Dependence in the Copula-Based Regression, 136
6.4 Skew-Normal Copula, 138
6.5 Inference of Directional Dependence Using Skew-Normal Copula-Based Regression, 144
6.6 Application, 147
6.7 Conclusion, 150
7 Non-Gaussian Structural Equation Models for Causal Discovery 153
Shohei Shimizu
7.1 Introduction, 153
7.2 Independent Component Analysis, 156
7.3 Basic Linear Non-Gaussian Acyclic Model, 158
7.4 LINGAM for Time Series, 167
7.5 LINGAM with Latent Common Causes, 169
7.6 Conclusion and Future Directions, 177
8 Nonlinear Functional Causal Models for Distinguishing Cause from Effect 185
Kun Zhang and Aapo Hyvärinen
8.1 Introduction, 185
8.2 Nonlinear Additive Noise Model, 188
8.3 Post-Nonlinear Causal Model, 192
8.4 On the Relationships Between Different Principles for Model Estimation, 194
8.5 Remark on General Nonlinear Causal Models, 196
8.6 Some Empirical Results, 197
8.7 Discussion and Conclusion, 198
PARTIII GRANGER CAUSALITY AND LONGITUDINAL DATA MODELING 203
9 Alternative Forms of Granger Causality, Heterogeneity, and Nonstationarity 205
Peter C. M. Molenaar and Lawrence L. Lo
9.1 Introduction, 205
9.2 Some Initial Remarks on the Logic of Granger Causality Testing, 206
9.3 Preliminary Introduction to Time Series Analysis, 207
9.4 Overview of Granger Causality Testing in the Time Domain, 210
9.5 Granger Causality Testing in the Frequency Domain, 212
9.6 A New Data-Driven Solution to Granger Causality Testing, 216
9.7 Extensions to Nonstationary Series and Heterogeneous Replications, 221
9.8 Discussion and Conclusion, 224
10 Granger Meets Rasch: Investigating Granger Causation with Multidimensional Longitudinal Item Response Models 231
Ingrid Koller, Claus H. Carstensen, Wolfgang Wiedermann and Alexander von Eye
10.1 Introduction, 231
10.2 Granger Causation, 232
10.3 The Rasch Model, 234
10.4 Longitudinal Item Response Theory Models, 236
10.5 Data Example: Scientific Literacy in Preschool Children, 240
10.6 Discussion, 241
11 Granger Causality for Ill-Posed Problems: Ideas, Methods, and Application in Life Sciences 249
Katrina Hlav ková-Schindler, Valeriya Naumova and Sergiy Pereverzyev Jr.
11.1 Introduction, 249
11.2 Granger Causality and Multivariate Granger Causality, 251
11.3 Gene Regulatory Networks, 254
11.4 Regularization of Ill-Posed Inverse Problems, 255
11.5 Multivariate Granger Causality Approaches Using l1 and l2 Penalties, 256
11.6 Applied Quality Measures, 262
11.7 Novel Regularization Techniques with a Case Study of Gene Regulatory Networks Reconstruction, 263
11.8 Conclusion, 271
12 Unmeasured Reciprocal Interactions: Specification and Fit Using Structural Equation Models 277
Phillip K. Wood
12.1 Introduction, 277
12.2 Types of Reciprocal Relationship Models, 278
12.3 Unmeasured Reciprocal and Autocausal Effects, 286
12.4 Longitudinal Data Settings, 293
12.5 Discussion, 304
PARTIV COUNTERFACTUAL APPROACHES AND PROPENSITY SCORE ANALYSIS 309
13 Log-Linear Causal Analysis of Cross-Classified Categorical Data 311
Kazuo Yamaguchi
13.1 Introduction, 311
13.2 Propensity Score Methods and the Collapsibility Problem for the Logit Model, 313
13.3 Theorem On Standardization and the Lack of Collapsibility of the Logit Model, 316
13.4 The Problem of Zero-Sample Estimates of Conditional Probabilities and the Use of Semiparametric Models to Solve the Problem, 318
13.5 Estimation of Standard Errors in the Analysis of Association with Adjusted Contingency Table Data, 322
13.6 Illustrative Application, 323
13.7 Conclusion, 326
14 Design- and Model-Based Analysis of Propensity Score Designs 333
Peter M. Steiner
14.1 Introduction, 333
14.2 Causal Models and Causal Estimands, 334
14.3 Design- and Model-Based Inference with Randomized Experiments, 336
14.4 Design- and Model-Based Inferences with PS Designs, 339
14.5 Statistical Issues with PS Designs in Practice, 347
14.6 Discussion, 355
15 Adjustment when Covariates are Fallible 363
Steffi Pohl, Marie-Ann Sengewald and Rolf Steyer
15.1 Introduction, 363
15.2 Theoretical Framework, 364
15.3 The Impact of Measurement Error in Covariates on Causal Effect Estimation, 369
15.4 Approaches Accounting for Latent Covariates, 372
15.5 The Impact of Additional Covariates on the Biasing Effect of a Fallible Covariate, 375
15.6 Discussion, 379
16 Latent Class Analysis with Causal Inference: The Effect of Adolescent Depression on Young Adult Substance Use Profile 385
Stephanie T. Lanza, Megan S. Schuler and Bethany C. Bray
16.1 Introduction, 385
16.2 Latent Class Analysis, 387
16.3 Propensity Score Analysis, 389
16.4 Empirical Demonstration, 391
16.5 Discussion, 398
16.5.1 Limitations, 399
PART V DESIGNS FOR CAUSAL INFERENCE 405
17 Can We Establish Causality with Statistical Analyses? The Example of Epidemiology 407
Ulrich Frick and Jürgen Rehm
17.1 Why a Chapter on Design?, 407
17.2 The Epidemiological Theory of Causality, 408
17.3 Cohort and Case-Control Studies, 411
17.4 Improving Control in Epidemiological Research, 414
17.5 Conclusion: Control in Epidemiological Research Can Be
Improved, 424
INDEX 433
Alexander von Eye, PhD, is Professor Emeritus of Psychology at Michigan State University. His research interests include statistical methods, categorical data analysis, and human development. Dr. von Eye is Section Editor for the Encyclopedia of Statistics in Behavioral Science and is the coauthor of Log-Linear Modeling: Concepts, Interpretation, and Application, both published by Wiley.